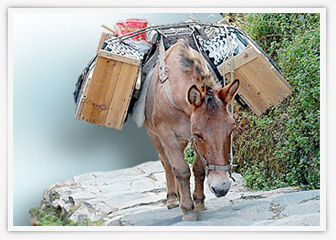
CLIENT
A Top 10 (by assets) internationally active US bank required to comply with the Basel II capital regime
CHALLENGE
In July of 2003, the Federal Reserve, the Office of the Comptroller of the Currency (OCC), the Federal Deposit Insurance Corporation (FDIC), and the Office of Thrift Supervision (OTS) jointly issued an Advanced Notice of Proposed Rulemaking (ANPR), a primer for U.S. implementation of the Basel II capital accord. The accord was originated by the Basel Committee on Banking Supervision (BCBS) and intended to harmonize best practices in risk-sensitive economic capital measurement with regulatory capital adequacy requirements. The ANPR outlined updated modeling options for both credit and operational risk, defining functionally advanced capital calculations. Within credit risk, the most advanced model, known as the Advanced Internal Ratings Based (A-IRB) approach, based capital on credit ratings crafted internally, evolving from the standardized ledger-based rules of Basel I, released in 1988, to a granular computation model, either at the customer account level or some aggregation thereof. The top 10 banks, with over $250 billion in total assets and internationally active asset portfolios of at least $10 billion, were notified of the expectation of compliance with A-IRB. Thus, their internal credit operations and data management capabilities would need upgrades where necessary to meet the requirements of compliance.
Notice of Proposed Rulemaking (NPR) succeeded ANPR in July of 2006, followed by final rulemaking in November of 2007. Implementation timelines were delayed as U.S. legislators weighed effects of lowered capital levels and competitive disadvantages to community banks lacking the infrastructure to support an advanced implementation, as well as wrangling over capital floors and transition periods in capital minimums from Basel I to Basel II. Final rulemaking called for parallel calculation of Basel I and II capital for a one year period, commencing sometime between 2nd quarter 2008 through a ceiling of 1st quarter 2010, with transitionary capital floors in place through three oversight periods following the end of parallel run.
And, that’s just U.S. rulemaking. For banks doing business outside of the U.S., they were under the same scrutiny to comply with interpretations of Basel II by foreign regulators, such as the Financial Services Authority (FSA) in the U.K. and the Australian Prudential Regulation Authority (APRA) in Australia. Thus, a bank with global reach would need to implement Basel II calculation and reporting solutions for each of the jurisdictions in which it operates, with each potentially on a different compliance timeline.
After years of uncertainty, the challenge facing the top 10 banks in the U.S. was finally crystallized by end 2007, as both foreign and domestic regulators had issued final interpretations of the latest Basel accord. As one of the top 10, our client was on center stage. Supported by a credit risk data warehouse platform for data management, their challenge lay in:
- Reviewing the calculation options within A-IRB by book of business and choosing approaches for U.S. compliance;
- Identifying the asset exposures originated in foreign jurisdictions and implementing an accepted calculation and reporting solution for those sub-portfolios;
- Conducting a fit/gap of their existing credit architecture against the chosen regulatory models, identifying data and design gaps, determining remediation steps, and implementing solutions;
- Upgrading their internal operations to support Basel identification, calculation, and model validation procedures.
Our client engaged the eBIS team to tackle challenges 2 & 3. Collaborating with a Big 4 partner responsible for items 1 & 4, we endeavored to comprehensively review Basel rulemaking and deliver solutions for jurisdictional compliance. And, we accepted perhaps one other challenge: prove the law of inertia, keeping a large initiative moving steadily forward with a push of positive momentum, overcoming the weight of modeling complexity and years of rulemaking uncertainty.
IDEAS
With a need for regulatory credit capital compliance both domestically and in foreign jurisdictions on divergent timelines, we saw an opportunity to approach the initiative in three distinct but complimentary parts: 1) domestic compliance, 2) foreign compliance and 3) infrastructure and solution maintenance upgrades. We set out to generate ideas in each area, which, taken together, could amount to a cohesive regulatory compliance plan.
Domestic Compliance
In 2008, there remained significant analysis to complete for domestic Basel compliance, and not much opening left in the window for parallel run. By the second quarter, some U.S. banks had already begun their one-year parallel run, while our client was in the throes of contemplating the cost/benefit of various A-IRB calculation methods by asset type. With limited time, we recognized the need to leverage the client’s existing architecture as much as possible. We recommended completing a comprehensive fit/gap against their credit risk data warehouse, a platform for both regulatory and economic capital management, once the calculation methods were chosen. With concurrence from the client, our eBIS team compiled the fit/gap analysis, identifying over 70 distinct data and modeling gaps against the selected A-IRB methods and the FFEIC reports required for Basel compliance. Along with identification of gaps, we also provided recommendations for remediation. A sampling of our ideas in key functional categories:
- Counterparty Scorecards for Probability of Default (PD) estimation: In a desire to migrate to a more quantitative factors-based rating model for wholesale counterparties, the client chose a scorecard solution from a ratings agency. The tool would allow through-the-cycle empirical data for stressed (economic downturn) parameter estimation. With a decisively stated rating philosophy as a Basel II requirement, we suggested management of the project with a resource familiar with the legacy credit system, one that relies on expert judgment to a greater degree. For, although the scorecard process would be decidedly empirical, expert judgment would still be necessary for counterparties that lack sufficient credit history or proxies.
- Historical reporting, model validation and Quantitative Impact Studies (QIS): Banks may estimate risk parameters, but what can determine their accuracy? Validating history against a once forward looking estimation is the Basel requirement. We recommended the creation of an history environment (see infrastructure section) to store all historical data for just this purpose. It would include loss recovery data (LGD validation), facility drawdown patterns (EAD validation), and historical counterparty defaults, under the Basel definition, for PD validation. In addition, Basel II rules include stipulations requiring visibility to the lineage of a customer relationship and its exposures, called “cradle to grave.” The archive solution would also facilitate such an analysis, showing the point at which the relationship began with the first approval of credit and all subsequent draws and changes in the level of credit extended. For QIS on demand, as is often requested by regulatory authorities during the rulemaking process, the archive would act as a sandbox for culling the specific historical data necessary for analysis. A custom interface could supply altered calculation parameters for investigation of how a new regulatory method would affect any subset of data.
- Pillar III reporting: Create a datamart to model capital calculations for public disclosure under Pillar III. Include the attributes necessary to model capital amounts and structure by legal entity or depository institution, with logic to analyze corporate structure and exclude or haircut subsidiaries not mandated for reporting. Design such that required capital levels, e.g., Tier 1 and 2 and total eligible, could be easily reported by dimension, e.g., asset category or RWA calculation, along with other metrics, such leverage ratios, including the compilation of non-risk weighted asset denominators where required.
- 3rd Party Guarantee modeling: We conducted an analysis of approved modeling options, comparing them to the data architecture available through their warehouse. We recommended the PD substitution approach, which, although differing from LGD adjustment in their economic model, would provide the path of least resistance for implementation. For, under the LGD adjustment method, the Basel accord calls for a comparison of capital required with LGD adjustment to that required with PD substitution, with LGD adjustment permitted only when it is punitive on a relative basis. Thus, LGD adjustment would require the PD substitution calculation, yet provide no capital benefit. To further ease implementation costs, we recommended disregarding partial guarantees and those with a maturity or currency mismatch.
- Credit Derivatives modeling: Credit derivatives posed a number of challenges. Purchased credit derivatives could qualify as synthetic securitizations and would need to be analyzed and identified. They also were not accounted for as a mitigant in ratings assignment. In regulatory calculations under the Current Exposure Method (CEM), counterparty credit risk is ignored under certain conditions, but reference asset risk is not, with capital required for sold credit derivatives. Finally, rules allow for a cap on potential future exposure (PFE) for these positions up to the amount of the unpaid premium. We recommended that they: a) establish internal process to analyze credit derivatives and tag their underlying assets as synthetic securitizations, which could feed into securitizations capital processing; b) update the LGD assignment process to include the effect of credit derivatives purchased as a guarantee; c) establish a process to analyze the 8 criteria for exclusion of counterparty risk on hedges, and tag the credit derivative exposures for such exclusion; d) for sold positions, establish a mapping from the credit rating of the reference asset to a defined PD risk rating, and model that mapped PD on the exposure; e) exlude the PFE benefit of the premium cap for the sake of implementation simplicity, which is allowed under the Basel concept of conservatism.
- Securitizations modeling: As with credit derivatives, the devil in securitizations compliance lies in the details. Of note, asset backed commercial paper (ABCP) support facilities would need to meet 4 criteria for preferable IAA treatment; quantification of exposure to purchased securitizations depends on accounting treatment (AFS vs. HTM); related securitizations can act as proxies for those that lack NRSRO ratings; the number of securities and seniority of purchased tranche affects capital computation. We recommended: a) an updated process for division portfolio managers to analyze ABCP support facilities and identify them as IAA eligible based on: the quality of the collateral pledged to the SIV, its investment guidelines, and an equivalent NRSRO rating for the SIV; b) using GL accounts to determine accounting treatment and unwinding unrealized gains and losses for purchased securitizations; c) improve securitizations workflow to analyze and identify candidates for proxy (subordination, no credit enhancements, equal or lesser duration); d) use a NRSRO to quantify positions in a securitization and a combination of asset class, payment factor, remaining maturity and credit rating to determine quality of the tranche purchased.
- Repo Style transaction modeling: For the largest portfolios, the bank chose a VaR approach, which was already in place and producing accurate results. For smaller portfolios, which had no validated VaR processing, an adjusted LGD approach was chosen. To facilitate accurate LGD assignment for these portfolios, in recognition of their over-collateralization and daily re-margining rules, we recommended two approaches: a) for those credits governed by facilities, enhance the LGD assignment process, managed by division portfolio managers, to consider the collateral rolling up to each facility; b) for those exposures with no credit facility, define the business practice for collateralization by exposure type and assign a default LGD rating representative of that collateral level. The default rating could be validated empirically and back tested through time against actual collateral levels and realized loss data.
Foreign Compliance
Foreign compliance centered on the FSA in the U.K., which permitted standardized computation methods, much akin to what was already in place for Basel I. To simplify modeling and lower implementation costs, the client chose this method. However, while the calculation methods were not new, the FSA reporting was mandated daily. Capital reports would need to be generated every business day by a stated “drop dead” time, within a required confidence interval.
Our idea was to tie this requirement to three infrastructure items: Service Level Agreement (SLA) adherence from providing systems, batch management, and external system integration. SLA adherence would monitor the timing, comprehensiveness, and quality of the U.K. data necessary for report generation, and manage exception causes with the source providers. Batch management would prioritize the technical jobs containing U.K. data so that they processed as soon as their dependencies were met, ahead of other competing jobs if necessary. External system integration would provide a mechanism to publish the data comprehensive of FSA report generation for consumption by the regulatory software vendor (see infrastructure section).
Infrastructure
The bank’s data warehouse platform was originally designed for a small scale profitability project, which expanded over time to reach quasi-enterprise warehouse scale. However, upgrades to the underlying infrastructure had not kept pace with functional demands. Terabytes of data were accumulating on a single production database, serviced by an outdated server. In addition, the environment served many masters from various functional areas, yet there was no tool to analyze the effect of a change in a technical object or piece of metadata on the array of applications that might use it. To address these back-end and operational constraints, we introduced a number of ideas:
- Infrastructure Upgrades: The production environment was behaving like a newborn baby. It demanded a lot of attention, but might still cry even after consoling. It was obvious to us that the infrastructure needed a thorough review, from servers to applications. We recommended performance studies on each tier of technology: a) hardware server architecture; b) operating system and network capacity; c) database configuration; d) application tuning. In addition, we recommended the creation of a test environment, mirroring the data load and configuration of the production environment, which would provide visibility to the effect of incremental application development. If new processing degrades performance, administrators could make proactive infrastructure changes before applications reach production.
- Historical Data Capture and Archiving: We suggested creating a separate environment for unlimited historical data capture, delineated temporally by data anticipated for reporting and older data that could be archived and analyzed by request. This environment for historical data capture and reporting would address a number of project requirements. First and foremost, it would provide the data needed for QIS on demand and credit parameter validation studies, both regulatory requirements. Secondly, such an environment would provide the fertile ground upon which robust reporting solutions could be built to feed internal analysis of credit risk, financial positions, and risk adjusted profitability. Finally, the environment would allow a migration and purge of historical data that was choking performance in the production database. A replication tool at the database level could port data across environments, making new data available in the history environment in near real time.
- Outbound System Integration: Providing data to other systems, particularly the Basel II regulatory reporting interface, presented some challenges. The data warehouse data model was not easily interpreted, with complex time dimensions and surrogate keys. In order to provide a comprehensive set of data from the most recent data load for a specific reporting date, it would prove useful to embed logic in a routine that executes within the warehouse and preps the data for consuming systems. Also, with system performance an issue, particularly select contention causing table locks, it was technically advantageous to publish data to tables configured specifically for outbound extraction. We recommended a publication solution to address these issues.
- Metadata Management: A data warehouse requires a patchwork of technologies to acquire, process, calculate, replicate, publish and report data. Normally each technology has its own metadata, or information about objects or data, to manage its domain of processing. However, no solution was in place in this environment to manage metadata across technologies, enabling users to analyze the universe of application technologies in a common format. We recommended the development of a metadata management solution for this purpose. Common metadata could also assist with change management, producing reports on the interconnectedness of objects and enabling users to understand how changes in one area affect downstream processing in separate, but related technologies.
SOLUTION
Many of the ideas that we presented were adopted, and we were instrumental in realizing them as solutions.
To stabilize their technical architecture, we managed improvements to their hardware, network, database, and applications. Of particular note, our analysis, advice and recommendations led to the acquisition of several new servers, reducing the processing strain in production and dedicating processing resources according to need: development, production, and historical reporting. We provided design oversight for the delivery of a metadata management tool, integrating information from multiple technologies for centralized analysis of linkages between technical objects. We designed and delivered a publication tool to lessen technical contention and present the right data for downstream Basel II capital calculations, report generation and consumption.
For foreign compliance, we implemented a data monitoring framework focused on SLA adherence, improving the timeliness of delivery by clearly defining data requirements and the locus of responsibility for data delivery. We also engineered a mechanism to prioritize the order of process execution and define dependencies for optimal efficiency.
For domestic rules adherence, we managed the implementation of the counterparty scorecard project, delivering a Basel-compliant wholesale customer scoring tool that was more in line with an industry best practice of modeling with quantitative factors. We designed, developed and managed the solution for historical reporting, credit parameter model validation and QIS, delivering a terabyte-scale data replication platform for near real-time data access. As experts on their data architecture and credit modeling, we provided advisory services on strategies for effective implementation of their favored A-IRB calculation methods.
In addition, we compiled and delivered functional training sessions, by asset category, on the chosen A-IRB modeling techniques under Basel II. The training sessions provided a financial interpretation of each group of exposures, a description of where related data originates and resides in the warehouse, the benefits of the chosen calculation model, the calculations performed, and the expected capital results, with every training participant receiving a training manual for ongoing reference. We also provided market commentary on why credit risk was relevant for each category of exposure, often submitting anecdotes relating the client’s portfolio to events that transpired during the global credit crisis.
RESULTS
Heading into 2008, our client faced high hurdles: standardized foreign compliance reporting, an effort to decipher and implement the final Basel II rules in the U.S. in a compressed time-frame, and stabilization of a temperamental technical platform supporting both initiatives.
Our solutions helped surmount all of these hurdles, contributing to efficient daily reporting to the FSA, a robust credit modeling architecture for domestic compliance, and a reliable platform on which to operate both. As a result, our client met its foreign reporting deadlines, and began its domestic Basel II parallel run by the required ceiling date of 1st quarter 2010.
After over 6 years of effort, the client had met all of its initial Basel reporting requirements. eBIS was there throughout, generating ideas and engineering solutions to help reach their Basel II compliance goals. The beast moved slowly and the load was heavy, but it walked consistently in the right direction with a concerted effort by both our client and its consulting partners.